Implicit neural representations for model reduction
New papers present an alternative view of kinematic approximation
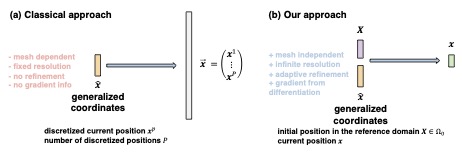
Excited to share a few of our recent research papers (with Maurizio Chiaramonte and our academic collaborators) that aim to unlock immersive Metaverse experiences via model reduction applied to large-scale computational-physics models. These papers show that leveraging implicit neural representations—which have been popularized in geometric computer vision (e.g., NeRF, DeepSDF)—brings many benefits when used to develop kinematic approximations for model reduction, such as facilitating analytic computation of spatiotemporal gradient and enabling simulation super-resolution.